In today’s world, there is a profound transformation happening in how we compete, create and capture value. With the speed at which technologies like Generative AI and Agentic AI are adopted widely, the whole relationship between humans and machines is getting redefined.
Quality engineering is no exception to this. Adoption of AI into the testing tools, Development and Testing of AI applications and development is on the rise with so many new tools and strategies emerging daily.
Enterprises are competing in the way they are running their entire quality engineering operation. Your competitor is running their QE operations with a team of one-third the size of yours without compromising the scale and accuracy. In fact, they are growing twice as fast as yours. How?
While most enterprises are still deploying likes of ChatGPT for generating content and creating chatbots, very few are fundamentally reimagining the quality engineering with AI. They are deploying the “Digital Tester”. These digital testers are nothing, but the digital teammate or digital colleague implemented using Agentic AI.
While these digital colleagues can drive quality engineering at incredible speed and scale, they also have their own unique characteristics and limitations. Understanding these characteristics not only guides us in terms of what tasks to delegate to these agents but also build a strong relationship that maximize the potential of both human and machines.
While these digital testers are evolving themselves from simple automation tools to the complex autonomous agents, it is very important to select the right digital tester depending on the various factors e.g. your use cases, technical complexity, implementation costs etc. It is very similar to onboarding a new team member and integrating him into your existing team.
Although it looks like a very simple choice, i.e. selecting the complex autonomous digital tester to reduce manual dependency and improve speed, it is a wise decision to opt for a digital tester which supports the entire continuum from automation to autonomy. This will allow flexibility so that we can use the different digital tester skills below as per the testing needs.
- Predictable and consistent behavior of digital testers with pre-defined rules; No learning and adaptation
- Digital tester leveraging LLMs for constraint awareness, but behavior is validated against predefined rules
- Digital tester with reasoning and action; Mult-step workflows are broken down into smaller actionable paths
- Digital tester’s Reasoning and action combined with RAG for external knowledge sources
- Integrate Digital tester with multiple tools for leveraging APIs and other software
- Self-reflecting /analyzing Digital tester using feedback loops
- Digital tester recalls relevant past experiences, preferences & uses this context for Reasoning
- Digital testers actively manipulate and control digital/physical environments in real time
- Digital testers improve themselves over time, learning from interactions, adapting to new environments, evolving
In its simplest terms, the testing needs of any enterprise can be broadly categorized into “What”, “How” and “When” of a software feature and Digital testers with the above skills can help us in all these aspects. AI assisted testing for “What” part of the testing e.g. AI pattern recognition helping the testers to know which parts of the application are likely problematic based on the analysis of the past test cases and historical data. AI powered testing for “How” part of the testing e.g. Self-Healing with AI ensuring the test cases remain valid when changes occur without manual intervention. And AI agents for testing for “When” part of the testing e.g. Self-learning AI with ability to spot unusual behaviour in the application by learning from each test cases they execute or independently exploring the application to discover unexpected issues.
Another most important consideration while selecting the Digital tester is deployment of the digital tester to test AI/ML systems. As AI and ML become more prevalent in our lives, it’s crucial to ensure these systems are thoroughly tested to work as intended.
While selecting your digital tester, make sure that the digital tester can overcome the challenges like being non-Deterministic, Lack of adequate and accurate training data, testing for bias, Interpretability and Sustained Testing and supports the critical aspects of AI systems testing like data curation & validation, algorithm testing, performance and security Testing and regulatory compliance e.g. compliance towards the country’s AI act.
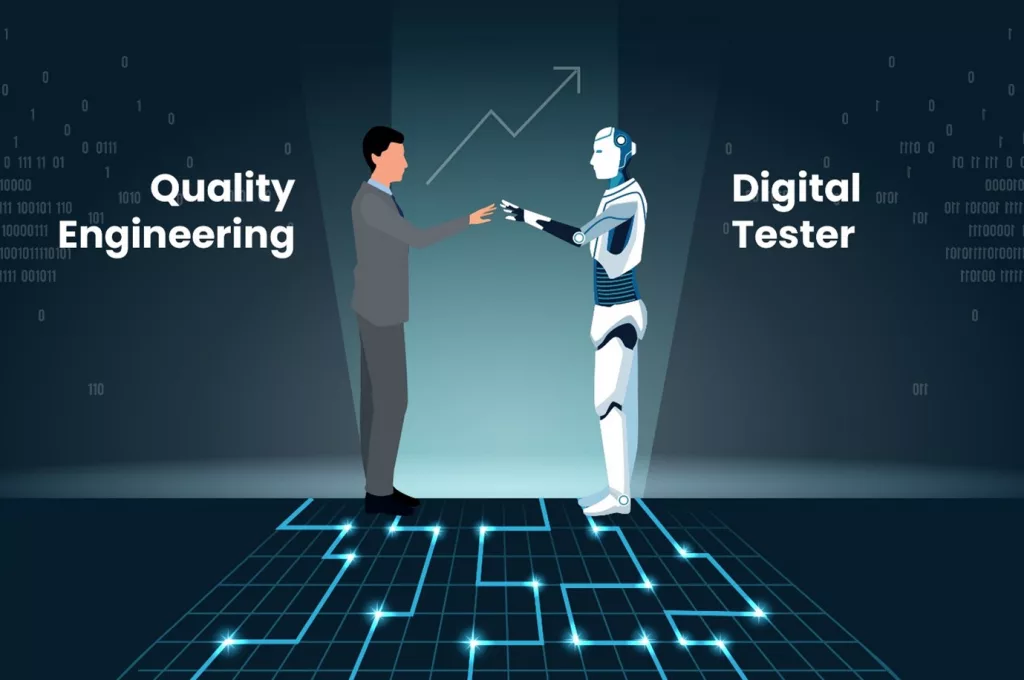
Summing Up
The rise of AI and Generative AI marks one of the most transformative shifts in our lives. Over the past decade, advancements in machine learning, deep learning and neural networks have driven artificial intelligence theoretical concepts into real worlds applications. This evolution has revolutionized quality engineering, where AI became an integral part of the traditional testing platforms and tools. These platforms no longer remained only tools, but they have evolved into a complete Digital Tester which can be part of your Quality Engineering team and work in collaboration with the humans to deliver an exceptional result. As businesses increasingly use AI to construct systems and applications, these Digital Testers are now in turn made to test the AI applications.
The AI testing approaches, procedures and platforms will continue to evolve and improve over the next few years, eventually approaching the maturity and standardization of Digital Testers in the quality engineering landscape.